How to Use AI and Machine Learning in Stock Market Trading?
Artificial Intelligence (AI) and Machine Learning (ML) are transforming the world of stock market trading by offering advanced tools and techniques that can enhance trading strategies, improve decision-making, and manage risks more effectively. In this comprehensive guide, we’ll delve into how AI and ML are applied in stock market trading, their benefits, challenges, and practical applications.
Understanding AI and Machine Learning in Stock Market Trading
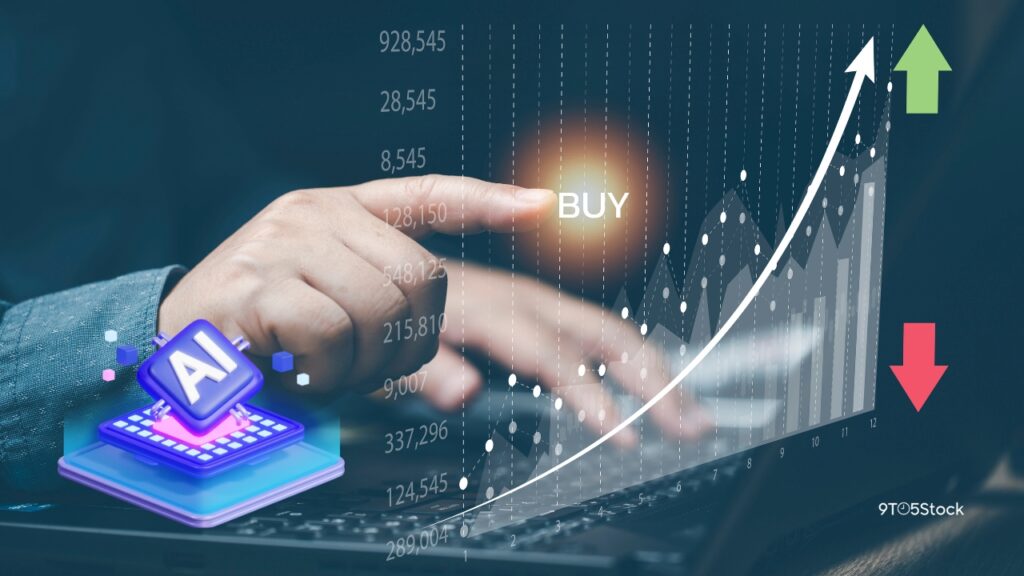
AI and machine learning have revolutionized various industries, including stock market trading. These technologies involve the use of algorithms and statistical models to analyze data, identify patterns, and make predictions. AI encompasses a broad range of techniques, including neural networks, natural language processing, and robotics, while machine learning focuses on enabling systems to learn from data and improve their performance over time.
AI and ML in stock trading allow for the automation of complex tasks, such as analyzing large datasets, recognizing market trends, and executing trades based on predefined criteria. This section explores the fundamental concepts of AI and ML and their applications in trading.
Also Read: Investing for Beginners: A Step-by-Step Guide
Key AI and ML Techniques Used in Trading
AI and machine learning employ various techniques to analyze and predict stock market behavior. Some key techniques include:
- Neural Networks: These are modeled after the human brain and are used for pattern recognition and prediction. They are particularly effective in handling large volumes of data.
- Decision Trees: These algorithms use a tree-like model of decisions and their possible consequences, including chance event outcomes.
- Support Vector Machines (SVM): SVMs are used for classification and regression tasks. They work by finding the hyperplane that best separates different classes in the data.
- Natural Language Processing (NLP): NLP helps in analyzing textual data, such as news articles and social media, to gauge market sentiment.
- Reinforcement Learning: This technique involves training models to make decisions by rewarding or penalizing them based on their actions.
These techniques are employed to build trading models that can predict stock prices, identify trading signals, and manage risks.
Advantages of Using AI and Machine Learning
The integration of AI and ML into stock market trading offers several advantages:
- Enhanced Accuracy: AI models can process vast amounts of data with high precision, leading to more accurate predictions and trading decisions.
- Automation: AI enables the automation of trading strategies, reducing the need for manual intervention and allowing for faster execution of trades.
- Risk Management: AI can help identify potential risks and anomalies in trading strategies, enabling traders to mitigate losses.
- Predictive Analytics: Machine learning models can analyze historical data to forecast future market trends and movements.
- Real-time Analysis: AI systems can analyze data in real-time, providing timely insights and enabling quick decision-making.
These benefits contribute to more efficient and effective trading strategies.
Challenges and Limitations
Despite its advantages, the use of AI and ML in stock market trading also presents challenges:
- Data Quality: AI models are highly dependent on the quality of data. Inaccurate or incomplete data can lead to flawed predictions.
- Complexity: Building and maintaining AI models can be complex and requires specialized knowledge and expertise.
- Overfitting: There is a risk of models becoming too tailored to historical data, leading to poor performance on new, unseen data.
- Regulatory Concerns: The use of AI in trading is subject to regulatory scrutiny, which can vary by jurisdiction.
Addressing these challenges is crucial for the successful implementation of AI and ML in trading.
How AI and ML Algorithms Are Developed?
Developing AI and ML algorithms for stock market trading involves several steps:
- Data Collection: Gathering relevant data, such as historical stock prices, trading volumes, and market indicators.
- Data Preprocessing: Cleaning and preparing the data for analysis, including handling missing values and normalizing data.
- Model Selection: Choosing appropriate algorithms and techniques based on the trading objectives and data characteristics.
- Training: Training the model using historical data to enable it to recognize patterns and make predictions.
- Evaluation: Assessing the model’s performance using metrics such as accuracy, precision, and recall.
- Deployment: Integrating the model into a trading system and monitoring its performance in real-time.
This process ensures that the AI models are effective and reliable for trading applications.
Case Studies of AI in Stock Market Trading
Examining real-world applications of AI in stock market trading provides valuable insights into its effectiveness. Some notable case studies include:
- Renaissance Technologies: Known for its Medallion Fund, Renaissance Technologies uses sophisticated AI and ML models to achieve exceptional returns.
- Two Sigma: This hedge fund employs machine learning algorithms to analyze market data and develop trading strategies.
- Goldman Sachs: Goldman Sachs uses AI for various trading and investment strategies, including high-frequency trading and risk management.
These case studies highlight the diverse ways in which AI can be leveraged for successful trading.
Also Read: Real Estate Investing: How to Build Wealth Through Property in 2024?
AI-Powered Trading Platforms and Tools
Several trading platforms and tools incorporate AI and machine learning capabilities. Some popular options include:
- MetaTrader 4/5: Offers plugins and tools that use AI for technical analysis and trading signals.
- TradeStation: Provides AI-powered analytics and trading automation features.
- AlphaSense: Utilizes AI for sentiment analysis and market research.
These platforms provide traders with advanced features and capabilities to enhance their trading strategies.
Best Practices for Integrating AI and ML in Trading
To maximize the benefits of AI and ML in trading, consider the following best practices:
- Start Small: Begin with a small-scale implementation and gradually scale up as you gain confidence and experience.
- Continuous Learning: Regularly update and refine your AI models to adapt to changing market conditions.
- Monitor Performance: Continuously monitor the performance of AI models and make necessary adjustments.
- Diversify Strategies: Use AI in conjunction with traditional trading strategies to diversify risk and improve overall performance.
Following these best practices can help ensure the successful integration of AI and ML into your trading operations.
Future Trends in AI and Machine Learning for Trading
The future of AI and ML in stock market trading is likely to be shaped by several emerging trends:
- Increased Automation: Greater automation of trading processes and decision-making.
- Enhanced Personalization: More personalized trading strategies tailored to individual preferences and risk tolerance.
- Integration with Big Data: Leveraging big data analytics to provide more comprehensive insights and predictions.
- Advancements in Natural Language Processing: Improved analysis of textual data for better sentiment analysis and market predictions.
Staying abreast of these trends can help traders stay competitive and make informed decisions.
Ethical Considerations in AI Trading
The use of AI in stock market trading raises several ethical considerations:
- Transparency: Ensuring transparency in AI models and decision-making processes.
- Bias: Addressing potential biases in AI algorithms that could impact trading decisions.
- Data Privacy: Protecting sensitive data and ensuring compliance with data privacy regulations.
Addressing these ethical considerations is essential for responsible AI use in trading.
Building Your Own AI Trading System
Developing a custom AI trading system involves several steps:
- Define Objectives: Clearly outline your trading goals and objectives.
- Gather Data: Collect and preprocess relevant market data.
- Develop Algorithms: Create and train AI models based on your trading strategy.
- Test and Refine: Test the system with historical data and refine it based on performance.
- **Deploy
**: Implement the system in a live trading environment and monitor its performance.
Building a custom AI trading system allows for greater control and customization according to specific trading needs.
AI and ML in Algorithmic Trading
Algorithmic trading relies heavily on AI and ML for executing trades based on predefined criteria. Key aspects include:
- High-Frequency Trading: AI algorithms can execute trades at high speeds and frequencies, taking advantage of minute price movements.
- Statistical Arbitrage: ML models identify and exploit pricing inefficiencies across different markets.
These applications demonstrate the power of AI and ML in optimizing trading strategies and improving efficiency.
Also Read: Unraveling the Mystery: How Interest Rates Shape Stock Market Outcomes?
Predictive Analytics and Forecasting in Stock Trading
Predictive analytics and forecasting are critical components of stock trading. AI and ML models use historical data to predict future market movements and trends. Techniques include:
- Time Series Analysis: Analyzing historical price data to forecast future prices.
- Regression Analysis: Identifying relationships between variables to predict market behavior.
Effective use of predictive analytics can enhance trading strategies and decision-making.
Backtesting AI Models
Backtesting involves testing AI models using historical data to evaluate their performance. Key considerations include:
- Historical Data: Using a comprehensive dataset to test the model’s accuracy and reliability.
- Performance Metrics: Evaluating metrics such as Sharpe ratio, drawdowns, and return on investment.
Backtesting helps validate the effectiveness of AI models and ensures they perform well under various market conditions.
Machine Learning for Risk Management
Machine learning can significantly enhance risk management in trading by:
- Identifying Risks: Detecting potential risks and anomalies in trading strategies.
- Predicting Volatility: Forecasting market volatility and adjusting strategies accordingly.
- Managing Portfolios: Optimizing portfolio allocation based on risk assessments.
Incorporating ML into risk management practices can lead to more robust and adaptive trading strategies.
Integrating AI with Traditional Trading Strategies
Combining AI with traditional trading strategies offers several benefits:
- Enhanced Decision-Making: AI provides data-driven insights that complement traditional analysis.
- Improved Efficiency: Automation and AI can streamline and enhance traditional trading methods.
Integrating AI with traditional strategies can result in a more comprehensive and effective trading approach.
Regulatory Issues and Compliance
Regulatory issues and compliance are crucial considerations when using AI in trading:
- Compliance with Regulations: Adhering to financial regulations and guidelines set by authorities.
- Transparency: Ensuring transparency in AI models and trading practices.
Navigating regulatory issues ensures that AI trading practices remain lawful and ethical.
Also Read: The Role of Robo-Advisors in Modern Investing
Learning Resources and Tools
To further explore AI and ML in stock market trading, consider the following resources:
- Online Courses: Platforms like Coursera and Udacity offer courses on AI and machine learning.
- Books: Titles such as “Machine Learning for Asset Managers” by Marcos López de Prado.
- Software Tools: Tools like TensorFlow, Keras, and scikit-learn for developing AI models.
These resources provide valuable knowledge and tools for mastering AI in trading.
Success Stories of AI in Trading
Successful implementations of AI in trading highlight its potential:
- DeepMind and AlphaGo: Demonstrated the power of AI in complex decision-making tasks.
- Numerai: Uses machine learning models submitted by data scientists to drive its hedge fund.
These success stories showcase the effectiveness of AI in achieving remarkable trading outcomes.
Getting Started with AI in Stock Market Trading
To begin using AI in stock market trading:
- Educate Yourself: Learn about AI and ML concepts and their applications in trading.
- Experiment with Tools: Explore AI trading platforms and tools to understand their capabilities.
- Start Small: Implement AI models on a small scale and gradually scale up as you gain experience.
Getting started with AI involves continuous learning and experimentation to achieve trading success.
Also Read: Gold and Precious Metals: Are They Worth Investing In? (2024)
Conclusion
The integration of AI and machine learning into stock market trading represents a significant leap forward in the evolution of trading strategies. By leveraging advanced algorithms and data-driven insights, traders can enhance their decision-making processes, improve accuracy, and manage risks more effectively. From predictive analytics and algorithmic trading to personalized strategies and real-time analysis, AI and ML offer powerful tools that can transform how trading is approached and executed.
However, while the advantages of AI in trading are substantial, it’s essential to navigate the associated challenges and ethical considerations carefully. Ensuring data quality, addressing potential biases, and adhering to regulatory requirements are critical for the successful and responsible use of AI in trading.
As technology continues to advance, staying informed about emerging trends and best practices will be crucial for traders looking to harness the full potential of AI and machine learning. By integrating these technologies with traditional trading strategies, traders can achieve a more comprehensive and effective approach, ultimately leading to better trading outcomes.
Whether you are a seasoned trader or just beginning to explore AI and machine learning, the insights and practices outlined in this guide will serve as a valuable resource for leveraging these technologies in stock market trading.
Disclaimer: The information provided in this article is for educational purposes only and does not constitute financial advice. Always consult with a financial advisor before making investment decisions.
Hello guys! My name is David Wilson, and I'm a passionate stock market enthusiast and the founder of 9to5Stock. With a deep understanding of market dynamics and a commitment to empowering others, I share valuable insights, strategies, and updates to help investors like you make informed decisions and achieve financial success. Welcome to our community, and let's thrive together in the world of investing!